The Environmental Impact of Training Large AI Models: The Need for Sustainable AI
- Jan 9
- 3 min read
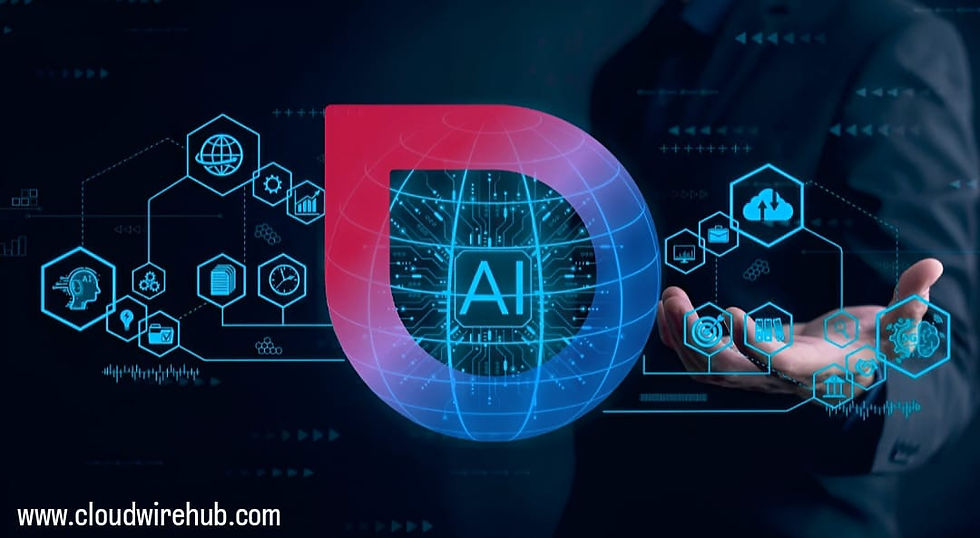
Training GPT-3 consumed an estimated 1,287 MWh of electricity, comparable to driving an electric car for 1.8 million miles.
As generative AI continues to revolutionize industries from transforming healthcare diagnostics to creating breathtaking artwork the spotlight on its benefits often overshadows its environmental costs. Behind the scenes, training and deploying large AI models such as GPT-4, DALL·E, and AlphaFold demand immense computational power, leading to significant energy consumption.
In this blog, we’ll uncover the environmental footprint of training large AI models, why it’s a critical issue, and innovative ways to make AI more sustainable. Let’s explore how cutting-edge technologies can achieve progress while preserving the planet.
The Hidden Cost of AI: Energy Intensive Training
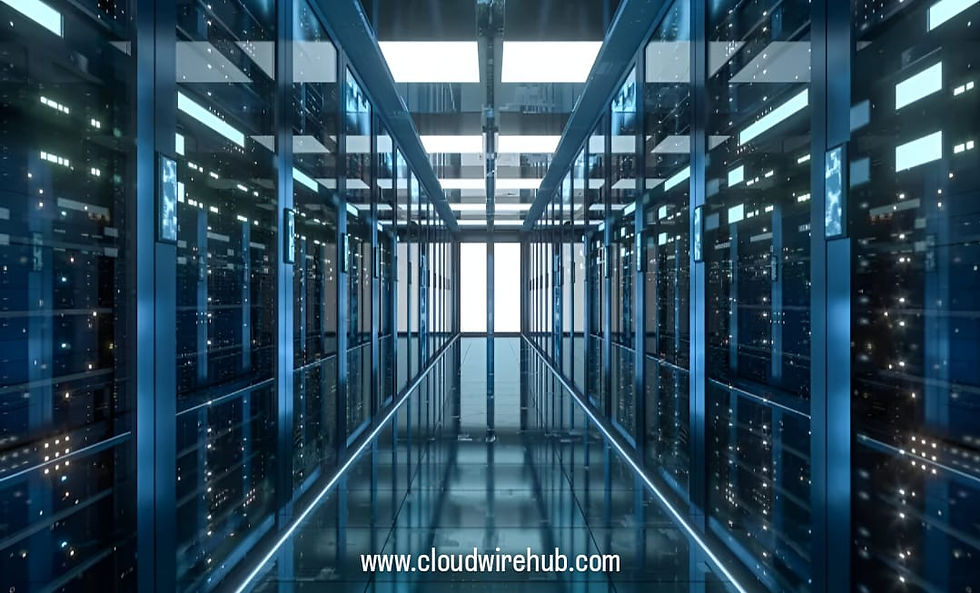
The world’s largest data center in Langfang, China, occupies 6.3 million square feet, nearly 110 football fields in size!
Training state of the art AI models is resource intensive. The architecture of models like GPT-4, BERT, and DALL·E comprises billions (or even trillions) of parameters, requiring extensive computational power, massive datasets, and prolonged training periods. These factors compound their energy consumption.
Key Factors Driving Energy Consumption
Data Processing and Supercomputing Clusters Training AI requires immense data processing capabilities, powered by GPUs (Graphics Processing Units) and TPUs (Tensor Processing Units). These devices consume enormous amounts of electricity.
Prolonged Training Durations Training GPT-3 took weeks and cost millions of dollars in energy expenses. GPT-4, with over a trillion parameters, likely exceeded these figures.
High-Performance Data Centers The hardware for training these models is housed in energy-intensive data centers with large cooling systems to maintain optimal performance.
The Carbon Footprint of AI: A Staggering Reality
A study by the University of Massachusetts Amherst revealed that training a large transformer-based model could produce 626,000 pounds of CO2 emissions, equivalent to the lifetime emissions of five cars. With GPT-3 boasting 175 billion parameters and GPT-4 surpassing 1 trillion, the environmental cost continues to escalate. These figures highlight the trade-off between innovation and sustainability, urging the tech community to find greener solutions.
The Impact of Model Size on Sustainability
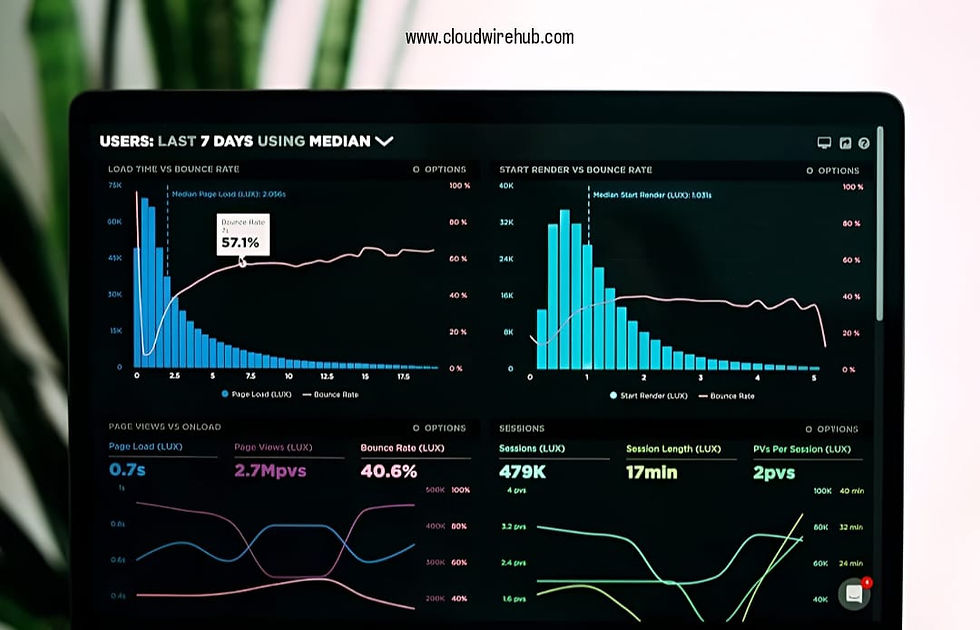
GPT-2 had 1.5 billion parameters, while GPT-4 likely exceeds 1 trillion, a size increase of over 666,667%!
The rapid growth of AI models has fueled remarkable progress but has also amplified environmental challenges:
GPT-2 (2019): 1.5 billion parameters
GPT-3 (2020): 175 billion parameters
GPT-4 (2023): Over 1 trillion parameters
Each leap in model size translates to exponential increases in energy usage. The race for bigger and better AI systems, while exciting, risks leaving a massive carbon footprint.
Can Generative AI Be Sustainable?
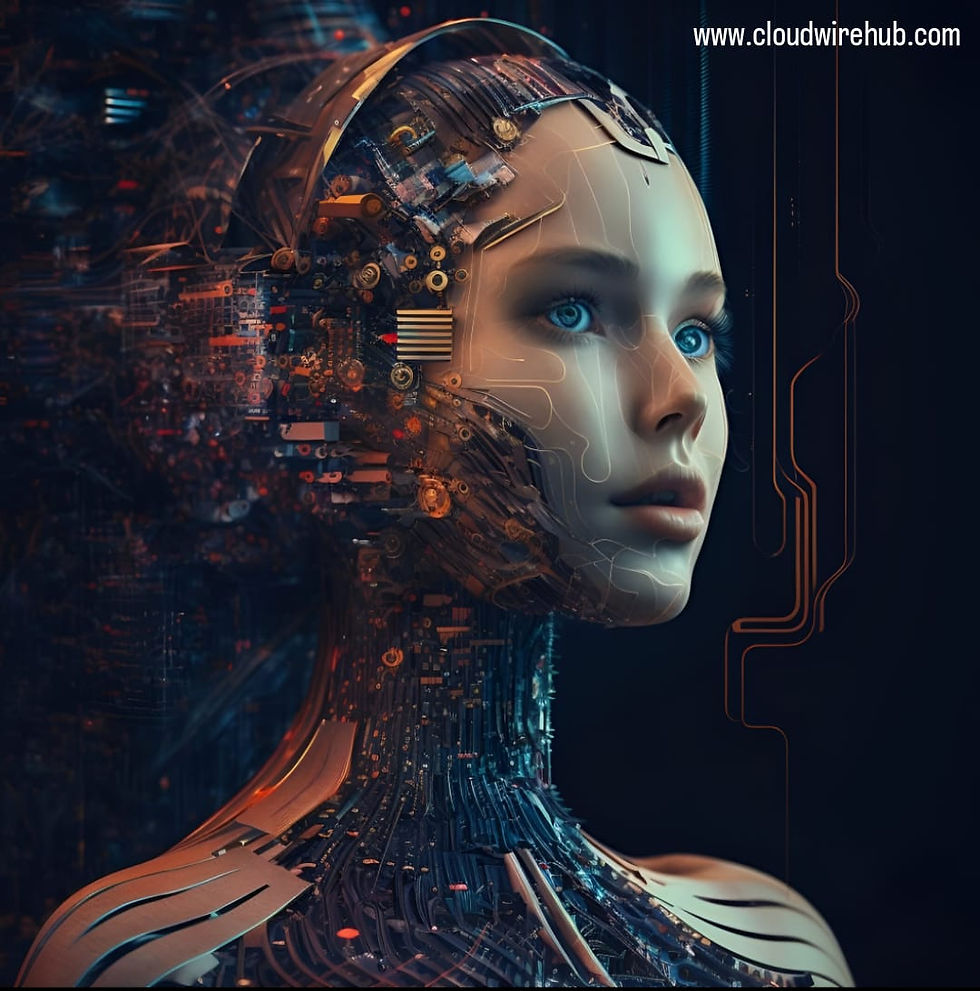
Pruning reduces the model’s size by up to 80%, drastically decreasing energy consumption without affecting accuracy.
Despite these challenges, sustainability in AI is achievable. The tech community is working towards solutions that balance innovation with environmental responsibility. Here are some promising pathways:
1. Efficient Algorithms and Model Optimization
Distillation: Training smaller models to mimic larger ones, reducing computational demands.
Sparse Architectures: Activating only essential parameters during training to save energy.
Early Stopping: Halting training when performance stabilizes to prevent wasted resources.
2. Carbon-Neutral Data Centers
Tech giants like Google and Microsoft are transitioning to renewable energy for their AI operations, aiming for carbon neutrality. This shift could dramatically reduce the environmental burden of AI.
3. Model Compression
Pruning: Removing less critical neural connections to make models more efficient.
Quantization: Using lower precision data representation to conserve memory and power.
The Role of Collaboration in Sustainability
AI practitioners can adopt collaborative frameworks to share resources. Open-source models like GPT-2 and T5 exemplify this trend, allowing smaller organizations to fine-tune pre-trained models instead of training from scratch.
What Can AI Practitioners Do?
Here’s how AI practitioners can reduce their environmental impact:
Use smaller, pre-trained models and fine-tune them for specific tasks.
Partner with cloud services powered by renewable energy.
Support research into energy-efficient AI algorithms and hardware.
Conclusion: Building a Sustainable AI Future
The environmental impact of training large AI models is undeniable, but it’s not insurmountable. With innovations in algorithm design, renewable energy-powered data centers, and collaborative practices, the AI community can lead the charge toward a greener future.
By embedding sustainability into the DNA of AI systems, we can continue to push technological boundaries without compromising the health of our planet. Together, we can create a future where generative AI thrives responsibly.
Comments