How to Used Machine Learning in Healthcare.
- Dhruv Patel
- Dec 7, 2024
- 3 min read
Updated: Jan 7

ML is one such phenomenon that integrates the practice of healthcare for novel ways in the analysis of health care information. A step-by-step guide on the utilization of ML with health information systems follows here.
Define the Problem

Examples: Predict disease outcomes, optimize resource allocation, personalize patient treatment, detect anomalies in medical imaging.
Collaborate with healthcare professionals to identify challenges that can benefit from ML solutions.
Personalized Care
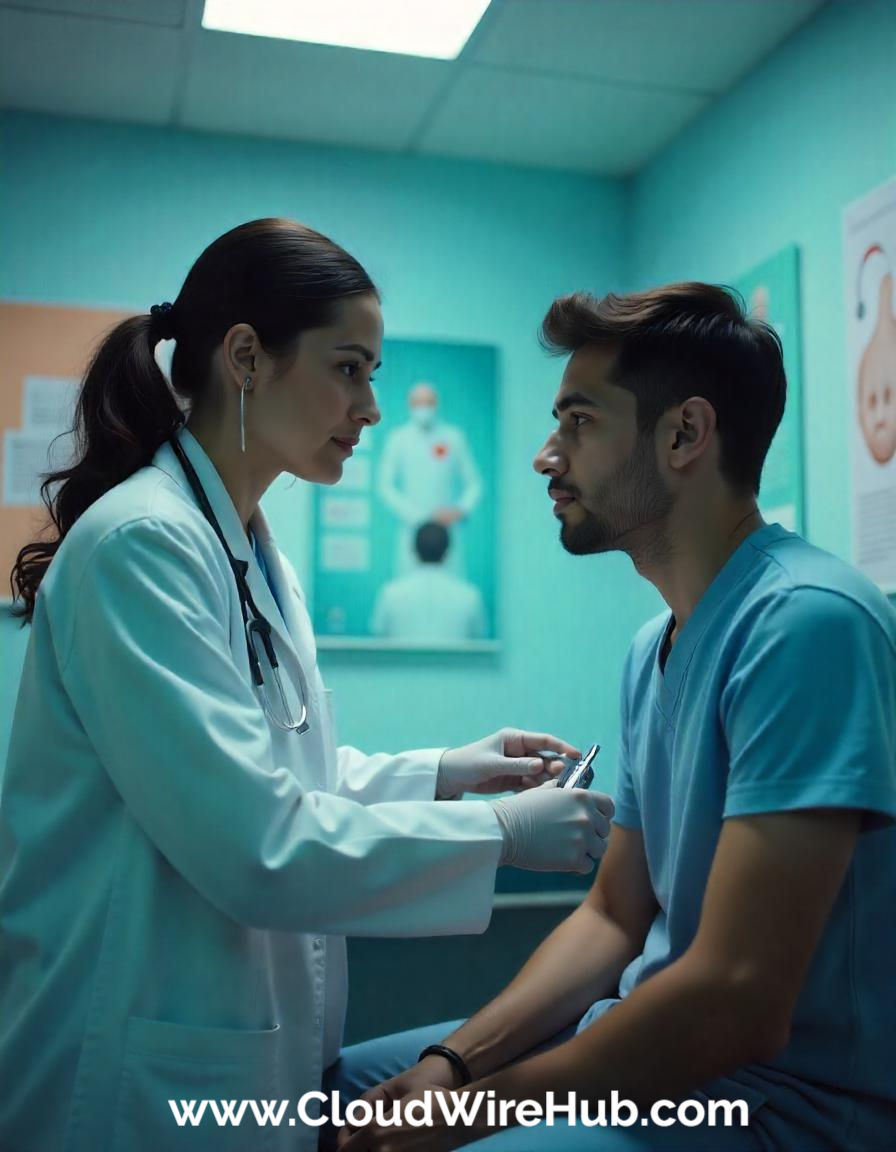
Through genetic information and history, ML can offer customized care plans for patients, ensuring a better outcome.
ML supports the prediction of the responses of patients to certain medications, which ensures precision medicine.
Drug Development and Discovery
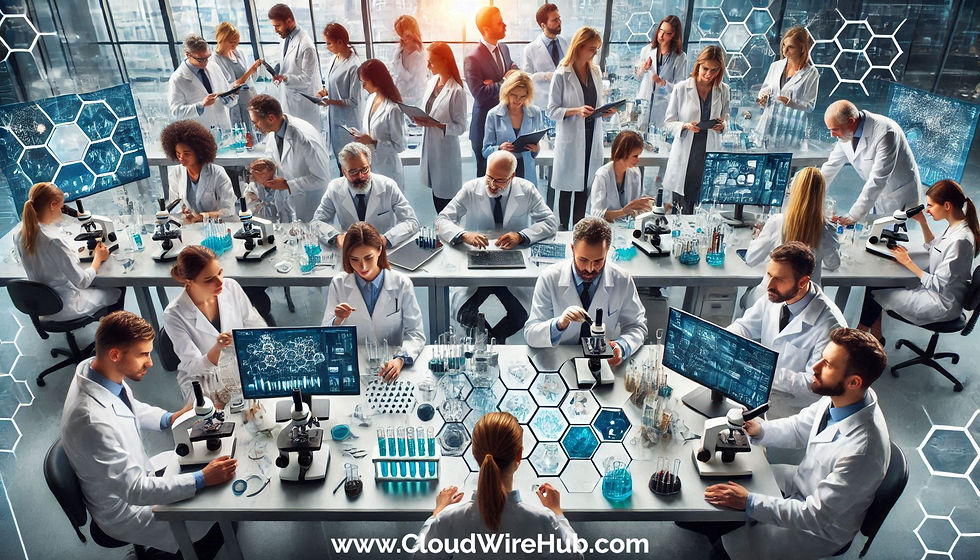
ML fast tracks the drug discovery process through the identification of potential compounds, forecasting their impact, and refining the clinical trials.
AI simulation saves time and cost on the drug development pipeline.
Healthcare Operations Optimization
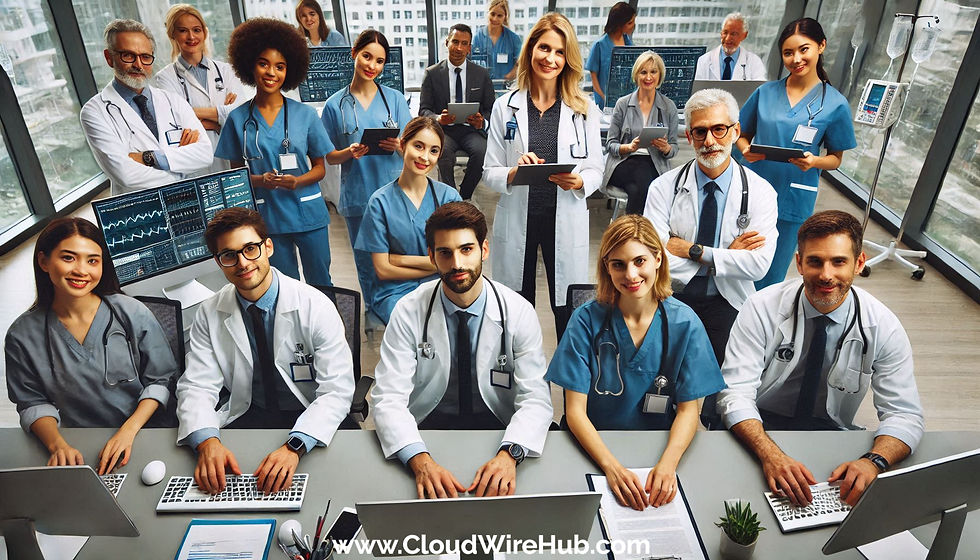
Predictive analytics optimize the operations in the hospitals by predicting admissions, personnel, and resources.
Chatbots and virtual assistants streamline interactions with patients and scheduling.
Remote Patient Monitoring
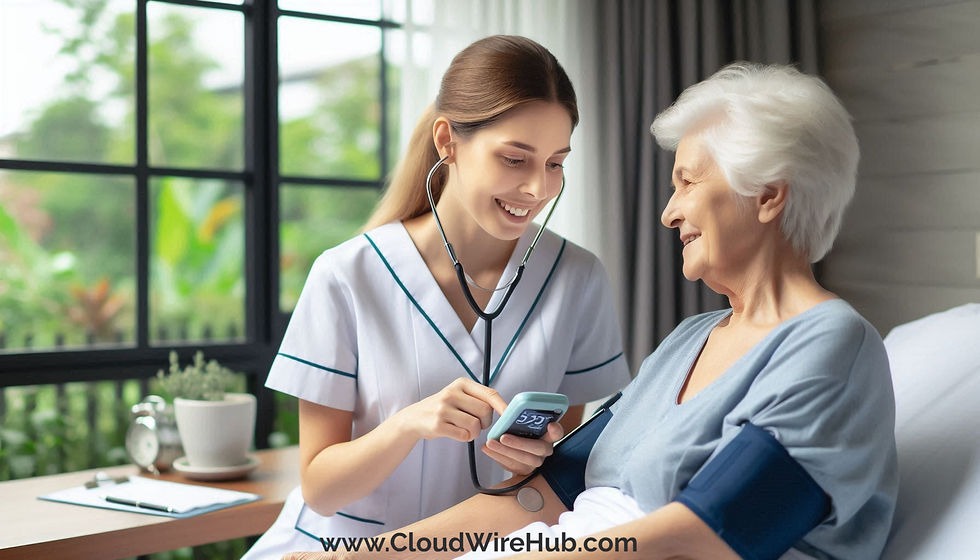
Wearable devices that integrate ML will be monitoring vital signs, for instance, heart rate, and glucose levels, thereby providing real-time detection of anomalies.
Among those, predictive models assess falls in elderly patients and deterioration in chronic conditions.
Healthcare Data Management
ML organizes and analyzes vast amounts of unstructured data, including electronic health records, for actionable insights.
NLP is used to interpret clinical notes, and documentation becomes streamlined.
Advantages of Machine Learning in Healthcare
Accuracy and Efficiency Improved
Lessens the error in diagnosis and yields results faster than the traditional processes.
2. Cost-Effective
Decreases the cost of clinical trials, operational inefficiency, and misdiagnosis.
Improved Patient Care
Allows personalized medicine, real-time monitoring, and early intervention to improve the outcomes of patients.
Scaling
Processes large-scale data, which helps solve global healthcare problems, such as disease outbreaks.
Continuous Learning
The algorithms learn with time, hence enhancing performance and keeping up with new medical discoveries.
Automation
Automated repetitive tasks such as administrative work so that more time in healthcare and care.
Key Considerations
Using machine learning (ML) in healthcare has transformative potential; its key features enable more accurate, efficient, and personalized medical care. Here are some of the core applications and features:
1. Diagnostic Support
Key Feature: Image Analysis ML models, especially convolutional neural networks (CNNs), are used to analyze medical images (e.g., X-rays, MRIs, CT scans) for detecting diseases like cancer, fractures, or organ abnormalities.
Example: Tumors, diabetic retinopathy, or lung diseases detection with radiological imaging.
Natural Language Processing (NLP)
NLP algorithms process patient notes and medical histories to offer diagnostic recommendations or patterns.
Example: Clinical note summaries for specific markers of disease.
Predictive Analytics
Key Feature: Risk Stratification
Patient outcome prediction, that is, the chances of developing chronic conditions, like diabetes and heart disease, based on patient data.
Example: Predicting hospital readmission or the progression of disease.
Epidemiological Predictions
ML models are trained on large-scale data to predict the spread of infectious diseases or the impact of public health interventions.
Example: Forecasting flu outbreaks or COVID-19 spread.
Patient Engagement
Key Feature: Wears Integration
ML reads information from wearables (smartwatches, fit bit) to give real-time health feedbacks.
Example: Arrhythmia detection using the smart watch ECG.
Behavioral Insights
Personalized health advice for healthier living
Example: Customized fitness and diet advice based on activity.
Data Privacy & Security: There should be strong measures to ensure the data of patients is secure and adheres to the regulations (HIPAA, GDPR).
Ethics and Bias: The fairness and equity in the ML models should be ensured to avoid biased healthcare delivery.
Interoperability: The system should be integrated with existing healthcare IT systems (e.g., EHR systems).
Conclusion
Machine learning is revolutionizing healthcare by providing tools for faster diagnoses, personalized treatments, and efficient operations. Challenges such as data privacy, bias, and integration with existing systems are still present, but the potential benefits of ML—such as cost savings, better patient outcomes, and advancements in medical research—make it a critical asset for modern medicine.
As technology continues to evolve, collaboration between healthcare professionals, data scientists, and policymakers will further unlock ML's potential in shaping a healthier future.
Comentários